Network Psychopathology: Leveraging Vulnerability and Simulation Modelling for Effective Mental Health Triaging
Tracks
Diamond Ballroom I: In-Person & Virtual via OnAIR
Wednesday, June 4, 2025 |
2:15 PM - 2:35 PM |
Overview
Jonathan Yap & Haikel Muhamad, National University of Singapore
Presenter
Mr Muhamad Haikel Bin Mohamed
Associate Director & Head, Staff Wellbeing & Engagement
National University of Singapore
Network Psychopathology: Leveraging Vulnerability and Simulation Modelling for Effective Mental Health Triaging
Abstract
PROBLEM STATEMENT:
Conventional methods of analysing self-report clinical questionnaires often treat symptoms as isolated, missing the complex interactions between them. This can possibly oversimplify the data, leading to missed insights into how certain symptoms drive overall mental health.
Additionally, existing reliance on norms and cutoffs can obscure the nuanced understanding of symptom interplay, potentially misrepresenting an individual's mental health status and treatment needs
OUR APPROACH:
We propose an alternative way of analysing pre-intake screening clinical questionnaire data.
Our innovative approach integrates network-based analysis with vulnerability scoring & simulations to uncover the intricate interactions between symptoms in self-report clinical questionnaires.
WHAT WE DID:
Using network science, we analysed a total of n=507 client responses from the Outcome Questionnaire 45 collected between 2022 and 2024 from staff seeking mental health services from our department.
Using a unique vulnerability formula that we developed, we identified key nodes that could inform targeted intervention. These nodes (see below) represent critical areas where disruptions can significantly impact the overall network, making them top targets for future interventions based on their vulnerability scores:
- I feel blue
- I am satisfied with my life (reverse-coded)
- I feel hopeless about the future
- I have trouble getting along with friends and close acquaintances
- I have trouble at work/school because of drinking or drug use
For example,
“I feel blue” -> possibly indicates emotional distress that may require mood-focused interventions for future sessions.
“I have trouble getting along with friends and close acquaintances” -> reflects social challenges that could exacerbate mental health issues; may require further social skills training.
Additionally, to validate the formula, we conducted an ablation intervention on our OQ-45 network to evaluate the impact of key components—degree centrality, betweenness centrality, and clustering coefficient—on vulnerability scores by systematically removing each variable and observing the effects.
Three Key Learnings:
1. Discover how network science can reveal hidden patterns in pre-intake clinical data, providing fresh insights into mental health.
2. Learn how bootstrapping can model the complexity of mental health symptoms, supporting more nuanced and effective intervention planning.
3. Discover how to create an RShiny app that automates data analysis, transforming your clinical data into network visualisations for real-time insights.
Conventional methods of analysing self-report clinical questionnaires often treat symptoms as isolated, missing the complex interactions between them. This can possibly oversimplify the data, leading to missed insights into how certain symptoms drive overall mental health.
Additionally, existing reliance on norms and cutoffs can obscure the nuanced understanding of symptom interplay, potentially misrepresenting an individual's mental health status and treatment needs
OUR APPROACH:
We propose an alternative way of analysing pre-intake screening clinical questionnaire data.
Our innovative approach integrates network-based analysis with vulnerability scoring & simulations to uncover the intricate interactions between symptoms in self-report clinical questionnaires.
WHAT WE DID:
Using network science, we analysed a total of n=507 client responses from the Outcome Questionnaire 45 collected between 2022 and 2024 from staff seeking mental health services from our department.
Using a unique vulnerability formula that we developed, we identified key nodes that could inform targeted intervention. These nodes (see below) represent critical areas where disruptions can significantly impact the overall network, making them top targets for future interventions based on their vulnerability scores:
- I feel blue
- I am satisfied with my life (reverse-coded)
- I feel hopeless about the future
- I have trouble getting along with friends and close acquaintances
- I have trouble at work/school because of drinking or drug use
For example,
“I feel blue” -> possibly indicates emotional distress that may require mood-focused interventions for future sessions.
“I have trouble getting along with friends and close acquaintances” -> reflects social challenges that could exacerbate mental health issues; may require further social skills training.
Additionally, to validate the formula, we conducted an ablation intervention on our OQ-45 network to evaluate the impact of key components—degree centrality, betweenness centrality, and clustering coefficient—on vulnerability scores by systematically removing each variable and observing the effects.
Three Key Learnings:
1. Discover how network science can reveal hidden patterns in pre-intake clinical data, providing fresh insights into mental health.
2. Learn how bootstrapping can model the complexity of mental health symptoms, supporting more nuanced and effective intervention planning.
3. Discover how to create an RShiny app that automates data analysis, transforming your clinical data into network visualisations for real-time insights.
Biography
Trained as a social worker, counsellor, adult educator and nurse, Haikel has served local and international communities. He has previously worked on capacity building, casework and counselling as well as disaster relief and peace-time missions with organisations like Singapore Red Cross and Mercy Relief.
Passionate about mental health, Haikel has developed and provided training in psychosocial support. In his work, he has also provided mentorship and guidance to psychological first aid trainers and facilitators. He aspires to empower individuals and communities in their journeys to achieve optimal mental wellness.
On any ordinary day, he enjoys a cup of teh tarik (milk tea) or bubble tea somewhere while gaming or reading.
Mr Johnathan Yap
Associate Psychologist
National University Of Singapore
Network Psychopathology: Leveraging Vulnerability and Simulation Modelling for Effective Mental Health Triaging
Abstract
PROBLEM STATEMENT:
Conventional methods of analysing self-report clinical questionnaires often treat symptoms as isolated, missing the complex interactions between them. This can possibly oversimplify the data, leading to missed insights into how certain symptoms drive overall mental health.
Additionally, existing reliance on norms and cutoffs can obscure the nuanced understanding of symptom interplay, potentially misrepresenting an individual's mental health status and treatment needs
OUR APPROACH:
We propose an alternative way of analysing pre-intake screening clinical questionnaire data.
Our innovative approach integrates network-based analysis with vulnerability scoring & simulations to uncover the intricate interactions between symptoms in self-report clinical questionnaires.
WHAT WE DID:
Using network science, we analysed a total of n=507 client responses from the Outcome Questionnaire 45 collected between 2022 and 2024 from staff seeking mental health services from our department.
Using a unique vulnerability formula that we developed, we identified key nodes that could inform targeted intervention. These nodes (see below) represent critical areas where disruptions can significantly impact the overall network, making them top targets for future interventions based on their vulnerability scores:
- I feel blue
- I am satisfied with my life (reverse-coded)
- I feel hopeless about the future
- I have trouble getting along with friends and close acquaintances
- I have trouble at work/school because of drinking or drug use
For example,
“I feel blue” -> possibly indicates emotional distress that may require mood-focused interventions for future sessions.
“I have trouble getting along with friends and close acquaintances” -> reflects social challenges that could exacerbate mental health issues; may require further social skills training.
Additionally, to validate the formula, we conducted an ablation intervention on our OQ-45 network to evaluate the impact of key components—degree centrality, betweenness centrality, and clustering coefficient—on vulnerability scores by systematically removing each variable and observing the effects.
Three Key Learnings:
1. Discover how network science can reveal hidden patterns in pre-intake clinical data, providing fresh insights into mental health.
2. Learn how bootstrapping can model the complexity of mental health symptoms, supporting more nuanced and effective intervention planning.
3. Discover how to create an RShiny app that automates data analysis, transforming your clinical data into network visualisations for real-time insights.
Conventional methods of analysing self-report clinical questionnaires often treat symptoms as isolated, missing the complex interactions between them. This can possibly oversimplify the data, leading to missed insights into how certain symptoms drive overall mental health.
Additionally, existing reliance on norms and cutoffs can obscure the nuanced understanding of symptom interplay, potentially misrepresenting an individual's mental health status and treatment needs
OUR APPROACH:
We propose an alternative way of analysing pre-intake screening clinical questionnaire data.
Our innovative approach integrates network-based analysis with vulnerability scoring & simulations to uncover the intricate interactions between symptoms in self-report clinical questionnaires.
WHAT WE DID:
Using network science, we analysed a total of n=507 client responses from the Outcome Questionnaire 45 collected between 2022 and 2024 from staff seeking mental health services from our department.
Using a unique vulnerability formula that we developed, we identified key nodes that could inform targeted intervention. These nodes (see below) represent critical areas where disruptions can significantly impact the overall network, making them top targets for future interventions based on their vulnerability scores:
- I feel blue
- I am satisfied with my life (reverse-coded)
- I feel hopeless about the future
- I have trouble getting along with friends and close acquaintances
- I have trouble at work/school because of drinking or drug use
For example,
“I feel blue” -> possibly indicates emotional distress that may require mood-focused interventions for future sessions.
“I have trouble getting along with friends and close acquaintances” -> reflects social challenges that could exacerbate mental health issues; may require further social skills training.
Additionally, to validate the formula, we conducted an ablation intervention on our OQ-45 network to evaluate the impact of key components—degree centrality, betweenness centrality, and clustering coefficient—on vulnerability scores by systematically removing each variable and observing the effects.
Three Key Learnings:
1. Discover how network science can reveal hidden patterns in pre-intake clinical data, providing fresh insights into mental health.
2. Learn how bootstrapping can model the complexity of mental health symptoms, supporting more nuanced and effective intervention planning.
3. Discover how to create an RShiny app that automates data analysis, transforming your clinical data into network visualisations for real-time insights.
Biography
Johnathan is an Associate Psychologist at NUS Health and Wellbeing, serving a university community of over 18,000 staff and 48,000 students. He has a strong interest in the intersection of data science and clinical psychology, focusing on how data-driven approaches can enhance mental health care through personalised, efficient, and impactful interventions.
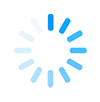